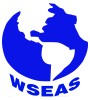
Plenary Lecture
Modeling and Change-Point Testing and Estimation of High-Dimensional Dependence Structures
Professor Ansgar Steland
Institute of Statistics
RWTH Aachen University
Germany
E-mail: steland@stochastik.rwth-aachen.de
Abstract: High-dimensional time series data play a crucial role in many areas such as biotechnology, engineering or environmetrics. Frequently, the question arises whether there are significant changes in the dependence structure of the variables, commonly described by the covariance matrix which is a difficult object in high dimensions. A common mathematical framework to model and analyze multivariate (high-dimensional) data streams are vector autoregressive moving average models with colored noise. These models allow to relate a set of variables to lagged values and, simultaneously, allow for (cross-sectional) correlations. A further class of models to described high-dimensional data are spiked covariance models. This talk presents a methodology suited for such models based on bilinear forms of the sample covariance matrix with respect to weighting vectors. It allows for analyses based on fixed projections, known bases (such as wavelets), random projections (have in mind the Johnson-Lindenstrauss theorem) or eigenstructures. The statistical procedures employ unweighted and weighted cumulated sum (CUSUM) statistics and associated change-point estimators, multivariate CUSUM transforms of growing dimension, in order to handle analyses in subspaces, and chisquare-tests. The asymptotic results are based on large sample approximations of partial sums and hold for a linear process framework without constraints on the growths of the dimension of the vector time series and of the CUSUM transform. The asymptotic variances and covariances of the cumulated sums can be estimated consistently by a class of homogeneous estimators. By studying consistency for sequential versions of these estimators, we are in a position to establish consistency of a stopped-sample estimator which segments the data and uses the pre-change segment for estimation. When using homogeneous estimators, the standardized CUSUM statistics allow for weak technical conditions l2-conditions on the projections. As a real world application sensor data from a large array of ozone monitoring stations is analyzed. Here the mean-corrected sensor data is compressed by projecting it onto sparse projections estimated from a learning sample.
Brief Biography of the Speaker: Ansgar Steland received the M.Sc. and Ph.D. degrees in mathematics from the University of Göttingen. After positions at Technische Universität Berlin, as a consultant, at the European University Viadrina and Ruhr-University of Bochum, he joined the faculty at RWTH Aachen University, where he was appointed Full Professor at the Institute of Statistics in 2006. He is an Elected Member of the International Statistical Institute and acts as the Chair of the Society for Reliability, Quality and Safety and as the Chair of the Section on Statistics in Natural Sciences and Technology of the German Statistical Society. Ansgar Steland organized and co-organized several international workshops and invited sessions, was member of program committees of several conferences and of a couple of prize committees. His main research interests are in change detection and quality control, high-dimensional statistics, time series analysis, nonparametric statistics, image analysis and applications to econometrics, natural sciences and engineering, especially photovoltaics.