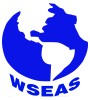
Plenary Lecture
Handling of Big Imbalanced Data Sets Classification Using Enriched over Sampling Techniques
Professors Sachin Subhash Patil
CSE Dept.
RIT Rajaramnagar & WCE Vishrambag
India
E-mail: sachin.patil@ritindia.edu
Abstract: Data generated in zettabytes per year has led a new avenue for its handling and analysis potentials. Facing this trial has triggered a vital role for new data management opportunities. NoSQL Big Data has stated on disseminating new test beds. It serves to determine huge unidentified standards from massive data sets. Additionally, handling classification of imbalanced Big Data sets (I.B.D.) has set a primacy alarm in the real world applications. It generally leads to incorrect results while using standard algorithms and classifiers. Similarly, misclassification cost and mixed class distribution tend to poor results. Non-binary class imbalance problem has drawn much attention in alignment to binary class. A novel data level non-clustered/clustered based solutions for enhanced over_sampling (O.S.) of minority instances are designed, implemented and planned to be discussed. They efficiently handle the classification of I.B.D. without mislaying valuable information. The proposed schemes are investigated for classification using various classifiers and validated using parameters as like F-measure, G-mean, ROC area. Projected techniques are executed using mapreduce environment on Apache Hadoop. The results signpost improved average scores of F-measure, G-mean and ROC area over various data sets from UCI repository. These techniques perform better on binary/non-binary class data sets compared to other traditional techniques (SMOTE, Safe-Level SMOTE, Borderline SMOTE, SMOTEBoost O.S. techniques). It helps to handle Big Data sets in size, varied imbalance ratio and an extreme number of attributes. Also, the planned Lowest v/s Highest (LVH) method, leverages the classification results of multi-class data sets compared to earlier One v/s All (OVA)/One v/s One (OVO) methods. The overall experimental outcomes attained, clearly evident the superiority of the offered schemes over the baseline schemes. Correspondingly, the projected techniques help to address fundamental data characteristics like small disjuncts, lack of density and overlapping.
Brief Biography of the Speaker: Sachin S. Patil was born in Mumbai, India, in 1981. He received the B.E. degree in computer science and engineering and M. Tech. in computer science and technology from the Shivaji University, Kolhapur in 2003 and 2011 respectively. He is pursuing a Ph.D. degree in computer science and engineering under A.I.C.T.E. Q.I.P. scheme at Walchand College of Engineering (Govt. aided and an Autonomous Institute) affiliated to Shivaji University, Kolhapur, MH India. Since 2010, he has been an Assistant Professor in the Computer Science and Engineering Department, Rajarambapu Institute of Technology, Rajaramnagar, MH – India. He has worked as head of Computer Science and Engineering department at Rajarambapu Institute of Technology, Rajaramnagar, MH – India. He is the author of a book chapter at Springer-Verlag and has more than 15 research papers. His research interests include Database Engineering and Big Data analytics. He has received a “Distinguished Facilitator” award at Inspire faculty contest organized by Infosys, Pune. He is a member of the IEEE.